Featured Research
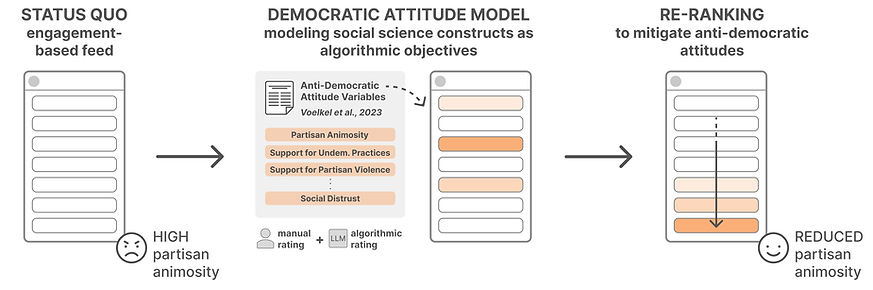
01
Embedding Democratic Values into Social Media AIs via Societal Objective Functions
In Proceedings of the ACM: Human-Computer Interaction (CSCW 2024)
Chenyan Jia*, Michelle S. Lam*, Minh Chau Mai, Jeffery T. Hancock, Michael S. Bernstein
Mounting evidence indicates that the artificial intelligence (AI) systems that rank our social media feeds bear nontrivial responsibility for amplifying partisan animosity: negative thoughts, feelings, and behaviors toward political out-groups. Can we design these AIs to consider democratic values such as mitigating partisan animosity as part of their objective functions? We introduce a method for translating established, vetted social scientific constructs into AI objective functions, which we term societal objective functions, and demonstrate the method with application to the political science construct of anti-democratic attitudes. Traditionally, we have lacked observable outcomes to use to train such models—however, the social sciences have developed survey instruments and qualitative codebooks for these constructs, and their precision facilitates translation into detailed prompts for large language models. We apply this method to create a democratic attitude model that estimates the extent to which a social media post promotes anti-democratic attitudes, and test this democratic attitude model across three studies. In Study 1, we first test the attitudinal and behavioral effectiveness of the intervention among US partisans (N=1,380) by manually annotating (alpha=.895) social media posts with anti-democratic attitude scores and testing several feed ranking conditions based on these scores. Removal (d=.20) and downranking feeds (d=.25) reduced participants' partisan animosity without compromising their experience and engagement. In Study 2, we scale up the manual labels by creating the democratic attitude model, finding strong agreement with manual labels (rho=.75). Finally, in Study 3, we replicate Study 1 using the democratic attitude model instead of manual labels to test its attitudinal and behavioral impact (N=558), and again find that the feed downranking using the societal objective function reduced partisan animosity (d=.25). This method presents a novel strategy to draw on social science theory and methods to mitigate societal harms in social media AIs.

02
Understanding Effects of Algorithmic vs. Community Label on Perceived Accuracy of Hyper-partisan Misinformation
In Proceedings of the ACM: Human-Computer Interaction (CSCW 2022)
Hyper-partisan misinformation has become a major public concern. In order to examine what type of misinformation label can mitigate hyper-partisan misinformation sharing on social media, we conducted a 4 (label type: algorithm, community, third-party fact-checker, and no label) X 2 (post ideology: liberal vs. conservative) between-subjects online experiment (𝑁 = 1,677) in the context of COVID-19 health information. We found that algorithmic and third-party fact-checker misinformation labels equally reduced people's perceived accuracy and believability of fake posts regardless of the posts’ ideology. We also found that among conservative users, algorithmic labels were more effective in reducing the perceived accuracy and believability of fake conservative posts compared to community labels. Our results shed light on the differing effects of various misinformation labels dependent on people’s political ideology.
Source Credibility Matters: Does Automated Journalism Inspire Selective Exposure?
In International Journal of Communication
To examine whether selective exposure occurs when people read news attributed to an algorithm author, this study conducted a 2 (author attribution: human or algorithm) × 3 (article attitude: attitude-consistent news, attitude-challenging news, or neutral story) × 2 (article topic: gun control or abortion) mixed-design online experiment (N = 351). By experimentally manipulating the attribution of authorship, this study found that selective exposure and selective avoidance were practiced when the news article was declared to be written by algorithms. For attitude-consistent gun-rights stories, people were more likely to expose themselves to human attribution stories rather than algorithmic attribution stories. This study bears implications on the selective exposure theory and the emerging field of automated journalism.

03
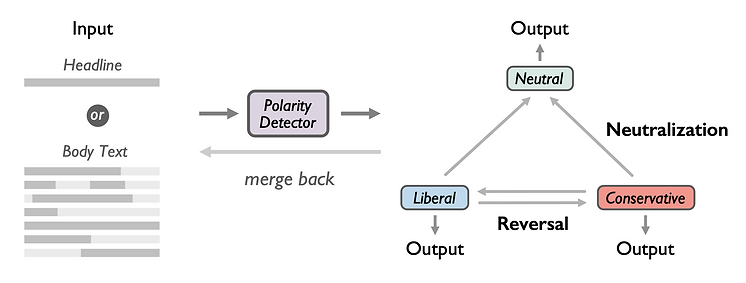
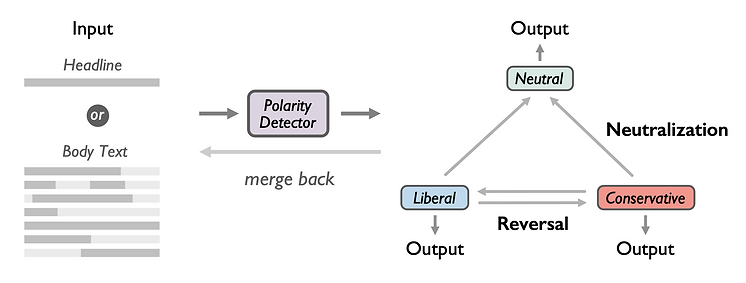
04
A Transformer-based Framework for Neutralizing and Reversing the Political Polarity of News Articles
In Proceedings of the ACM: Human-Computer Interaction (CSCW 2021)
We propose a computer-aided solution to help combat extreme political polarization. Specifically, we present a framework for reversing or neutralizing the political polarity of news headlines and articles. The framework leverages the attention mechanism of a Transformer-based language model to first identify polar sentences, and then either flip the polarity to the neutral or to the opposite through a GAN network. Tested on the same benchmark dataset, our framework achieves a 3%-10% improvement on the flipping/neutralizing success rate of headlines compared with the current state-of-the-art model. Adding to prior literature, our framework not only flips the polarity of headlines but also extends the task of polarity flipping to full-length articles. Human evaluation results show that our model successfully neutralizes or reverses the polarity of news without reducing readability. Our framework has the potential to be used by social scientists, content creators and content consumers in the real world.
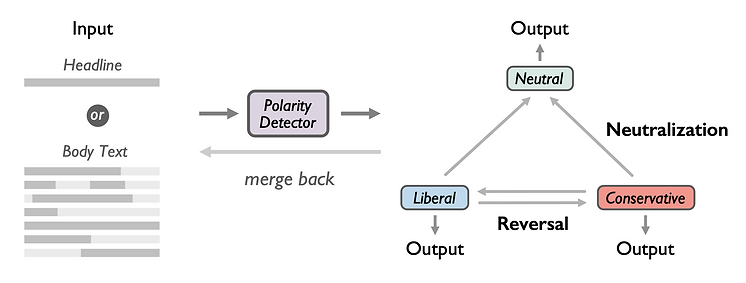
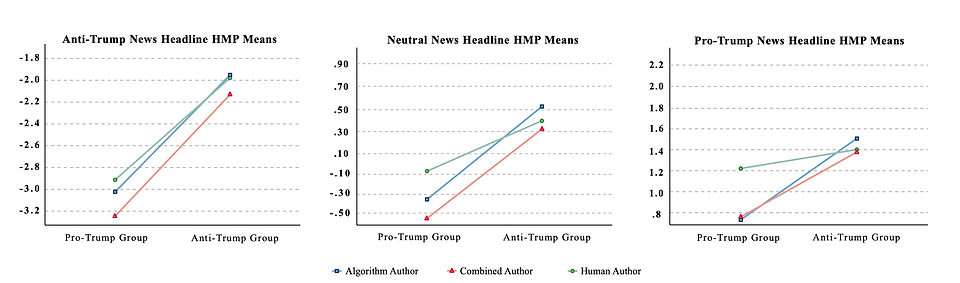
Algorithmic or Human Source? Examining Relative Hostile Media Effect With a Transformer-Based Framework
In Media and Communication
05
The relative hostile media effect suggests that partisans tend to perceive the bias of slanted news differently depending on whether the news is slanted in favor of or against their sides. To explore the effect of an algorithmic vs. human source on hostile media perceptions, this study conducts a 3 (author attribution: human, algorithm, or human-assisted algorithm) x 3 (news attitude: pro-issue, neutral, or anti-issue) mixed factorial design online experiment (N = 511). This study uses a transformer-based adversarial network to auto-generate comparable news headlines. The framework was trained with a dataset of 364,986 news stories from 22 mainstream media outlets. The results show that the relative hostile media effect occurs when people read news headlines attributed to all types of authors. News attributed to a sole human source is perceived as more credible than news attributed to two algorithm-related sources. For anti-Trump news headlines, there exists an interaction effect between author attribution and issue partisanship while controlling for people’s prior belief in machine heuristics. The difference of hostile media perceptions between the two partisan groups was relatively larger in anti-Trump news headlines compared with pro-Trump news headlines.
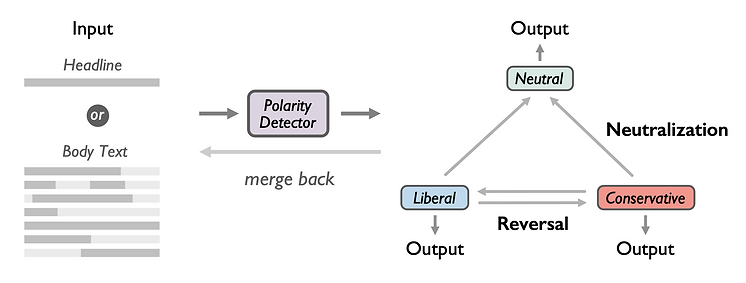
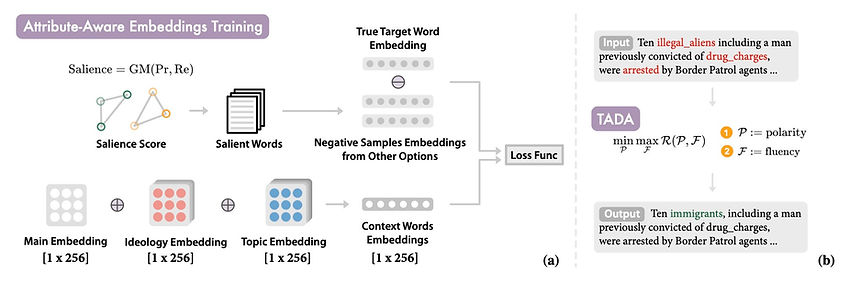
06
Political Depolarization of News Articles Using Attribute-aware Word Embeddings
In The Proceedings of the 15th International AAAI Conference on Web and Social Media (ICWSM 2021)
Ruibo Liu, Lili Wang, Chenyan Jia, Soroush Vosoughi
Political polarization in the US is on the rise. This polarization negatively affects the public sphere by contributing to the creation of ideological echo chambers. We introduce a framework for depolarizing news articles. Given an article on a certain topic with a particular ideological slant (eg., liberal or conservative), the framework first detects polar language in the article and then generates a new article with the polar language replaced with neutral expressions. To detect polar words, we train a multi- attribute-aware word embedding model that is aware of ideology and topics on 360k full-length media articles. Then, for text generation, we propose a new algorithm called Text Annealing Depolarization Algorithm (TADA). TADA retrieves neutral expressions from the word embedding model that not only decrease ideological polarity but also preserve the original argument of the text, while maintaining grammatical correctness. Our work shows that data-driven methods can help to locate political polarity and aid in the depolarization of articles.